Automating certain patient flows, like signposting patients to the right level of care can enable effective care and cover patient needs. Before contacting healthcare, most patients have a picture of their condition. This mindset can be of great benefit to both healthcare providers and patients, as the patients’ knowledge of their condition can be collected and aggregated before the medical consultation. But to automate that flow, we should ask what type of data is appropriate to build such a solution and how can this data be validated and quality assured in a way that ensures the safety of the patients?
In our previous article, we identified 3 requirements that are essential for the long-term use of an automated triage and anamnesis solution:
- patient-friendly communication
- awareness of the medical context
- integration into the digital-physical flow
In this article, we focus on the technical requirements of an automated solution for triage and anamnesis – the essentials that should be fulfilled in order to meet present and future needs. To develop an AI application, we need data. The type of data, as well as the collection of data, are largely dependent on what one wants to achieve with an automated solution. Different data sources have different advantages and limitations.
One possible source of data is historical data, collected from years of medical records. The advantage of this type of data is that it is collected over a long time and can encompass large amounts of information. Historical data is therefore well suited in areas such as radiology, where images of comparable quality can be reused. However, the application is more difficult in primary care settings where historical data are rarely homogeneous enough to be adapted to automated medical and triage solutions.
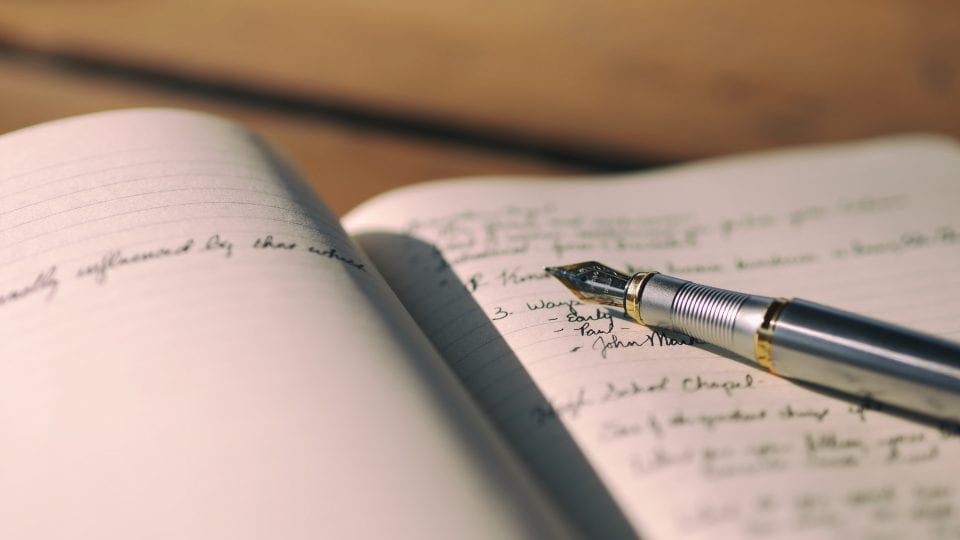
The second type worth considering is newly generated data. This can consist, for example, of logs from different tools that have been collected to process and compile data for different purposes. This type of data is often more homogeneous, but requires a lot of work and especially time to collect. It has to also be placed into context before it can be used. Additionally, it is vital to consider which data records are actually needed before starting to collect larger quantities. For that reason, it is important to strike a balance and be pragmatic enough to identify what type of data is sufficient and how it can be useful for the specific purpose. In the context of primary care, it would take several decades to obtain a comprehensive database for all types of conditions, with statistical relevance for different age groups, diseases, biological genders, etc. However, this type of data is well suited for validating models in segments where there is sufficient data density (eg in upper respiratory tract infections and stomach upset).
There is a third type of data that is frequently overlooked in AI applications, particularly in healthcare: Expert domain knowledge. This valuable resource should not be forgotten, especially in primary care, where there are enormous volumes of accumulated knowledge describing the relationship between symptoms and diagnoses. So why not reuse this knowledge? This knowledge is based on patterns and relationships that have been observed and analysed for thousands of years. Collecting this knowledge again and in a statistically relevant way would take decades of a tremendous amount of work. It’s a great gain to start with the relationships already described in the literature by medical experts and then refining them when enough additional data is collected. Nevertheless, a challenge with this type of source is that the knowledge needs to be translated into relevant data structures, which requires cross-functional collaboration between data engineers and medical personnel.
The type of available data, as well as its quantity and quality, will greatly affect how the automated solution can be applied and developed. But it is also critical to choose the right type of AI application, ie. the right kind of method to process the data source, for what you want to achieve – both today and tomorrow. In our next blog article on AI in primary care, we take a closer look at three common AI applications and deep dive into the method we have chosen to focus on in developing our automated triage and medical history solution, Red Robin. Read more here!